
Out-performing human capability, deep learning analytics engines can analyze vast amounts of data points taken from video footage across single or multiple cameras, covering an area, a site or multiple facilities simultaneously. Image courtesy of IDIS
Eyeing the Evolving Landscape of AI Surveillance
Explore trending benefits, systems integration insights, a challenges assessment, the impact of Generative AI on shaping the next generation video security, and more.
The adoption of artificial intelligence (AI) and its subsets has ushered in a new era of security innovation, particularly in the realm of video surveillance. From trend-setting benefits to heightened system effectiveness, AI is becoming a transformative force in fortifying infrastructure while delivering newfound business efficiencies.
SDM tapped the subject matter expertise of seven video security specialists to delve into the possibilities of AI-enabled applications. From real-time anomaly detection to predictive analytics, AI instills a level of intelligence that is revolutionizing traditional surveillance approaches. Ahead, we explore system integration and compatibility issues; assess challenges and threats; and shed light on evolving strategies as well as adaptive security solutions.
We also cast a spotlight on the impact of Generative AI, a force propelling video security into the realm of unparalleled sophistication. From its impact on behavior analysis to resolution enhancement, Generative AI represents the vanguard of next-gen surveillance.
Trending Benefits & Effectiveness
Designing a surveillance system is no longer as simple as mounting a camera and having it point in the right direction. Tara Dunning, vice president of global security, Wesco, Pittsburgh, PA, explains that advanced video analytics, powered by AI and deep learning, have transformed the surveillance camera into a powerful sensor able to more accurately and quickly detect threats.
“AI-powered video surveillance systems can improve threat detection and response by identifying threats such as intruders, weapons and suspicious activity more accurately and quickly than human operators,” she says. “This can help security personnel respond to incidents more effectively and reduce risks to the given environment.”
An obvious benefit of AI is enhancing video analytics and making them more reliable. Now, the many video analytics applications that have been around for a long time become feasible when adding machine learning (ML) to them, says Florian Matusek, director of video analytics, Genetec, Montreal.
“We see a lot of demand to solve perimeter protection scenarios, where video analytics is leveraged to detect intruders who breach the perimeter,” he says. “Video analytics systems can not only detect the intruder but also place the object on a map to allow tracking across cameras and to combine it with other sensors such as radar.”
The marketplace is also seeing a surge in people-counting and occupancy analytics applications, Matusek explains, as enterprises seek to understand better how their space is being utilized.
“By measuring the occupancy of spaces, many valuable business insights can be derived,” he continues. “How are my meeting rooms being used? Are large meeting rooms booked for small meetings? How much time do my employees spend waiting in line at the cafeteria and can I improve flow? How many shared desks are free per floor?”
AI-enabled surveillance systems are built on deep learning models with extensive real-world datasets and are trained to recognize, classify and locate humans and objects the way a human brain works. This is backed by powerful data processing capabilities that surpass what even a fleet of human brains cannot achieve simultaneously, explains Seetha Sasikala, senior global manager, global product solutions management, Teledyne FLIR, Wilsonville, OR.
“Such an AI integrated surveillance system actively supports site security operators by delivering situational awareness backed by real-time data, detection of real threats at early stages, filtering out perceived threats and alerting for quick remote interventions,” she says. “Furthermore, AI enables forensic research and analysis faster than ever before.”
Sasikala describes a particular use case where key benefits can be achieved by incorporating AI into video surveillance systems. With the increasing need for perimeter security and around-the-clock surveillance, business infrastructure and asset managers face two critical challenges — that of delivering efficiency and decision support.
This is where AI integration in video surveillance offers the biggest positive impact. Manned 24/7 operations not only add running costs, she says, but also cannot fully address the needs for long distance perimeter coverage.
“This is addressed by AI integrated thermal imaging sensor devices that can see and sense what humans cannot at long distances and in difficult-to-view, low-light outdoor conditions,” Sasikala says. “AI-powered surveillance devices are capable of processing large amounts of data and delivering precise, actionable input within seconds or minutes, where the same task can take hours for human investigation.”
Quang Trinh, business development manager, platform technologies, Axis Communications, Chelmsford, Mass., points out that adding AI tools and techniques to video surveillance systems drastically reduces false alerts and events. Current video analytics technologies are either pixel-based or event-based and when they are used in the real world, many uncertainties are introduced that cause the reliability of these systems to fluctuate.
“AI techniques such as deep learning significantly improve the detection and classification of people, vehicles and other critical features of an object that reduce many of the existing false alerts,” says.
Improving the efficiency of event detection and alerts is one of the primary benefits of AI in surveillance systems, Trinh adds. Automation is another important benefit, where data from video and audio systems, access control and building intrusion systems can be fused to work together through AI techniques in ML models in order to detect anomalies tailored to the customer’s environment.
“As the system becomes more responsive and filters out only what is critical, the system’s operator now has immediate information to act on, potentially reducing the time to respond to an incident and improving the safety and security of the organization,” Trinh continues.
Traditionally, the use of AI in video surveillance has primarily aimed to overcome the limitations of human monitoring and assessment of video scenes, explains Barry Norton, vice president of research, Milestone Systems, Beaverton, Ore. Humans experience fatigue, have limited information processing capacity and often struggle with comprehending complex patterns.
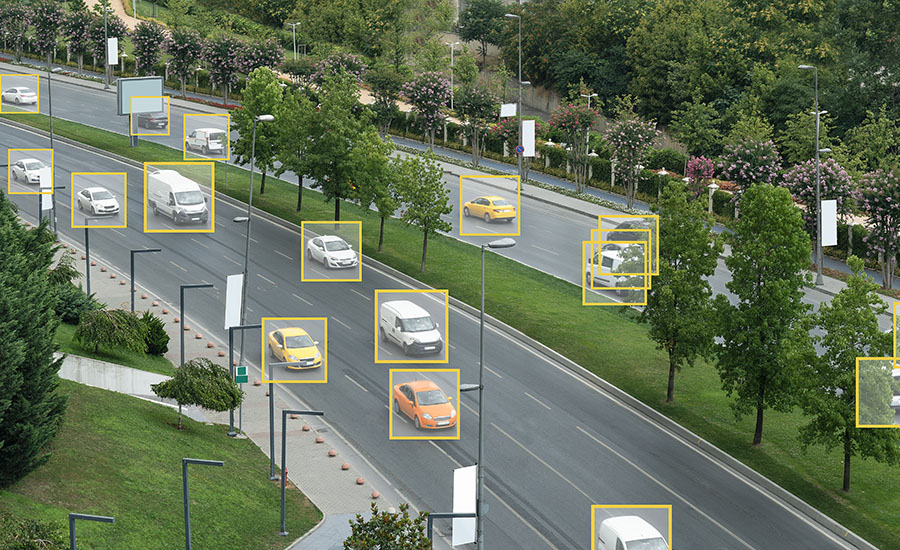
“However, AI solutions now offer a range of stronger and more valuable benefits that extend beyond these conventional techniques. Advanced AI-driven techniques in video surveillance have revolutionized security and protection,” Norton says. “They surpass mere object detection in video scenes; AI solutions can now identify and classify objects, track their movements, understand their interactions and even predict potential incidents before they occur.”
By leveraging multimodal techniques, AI solutions can combine video data with other types of data to extract actionable insights for humans Norton adds. “This strategic shift in security and protection is transforming video surveillance from a passive observer to a proactive tool for intelligent action.”
While noting game-changing benefits and newfound efficiencies realized with the application of AI in the past couple of years, Jason Burrows, sales director, IDIS America, Coppell, Texas, says 2023 “was the year AI really came of age.”
The mainstream conversation and debate around AI following the launch of OpenAI’s ChatGPT chatbot toward the end of 2022 benefited the security industry as end users and integrators became more confident about the benefits and accuracy of AI video, Burrows explains. This led to the discovery of new applications beyond just security across their organizations.
“A global survey we conducted throughout the summer [in 2023] across the security buying chain reflected this as well,” he adds. “It showed that just under 70 percent of those who operate or install surveillance solutions were using some form of AI in their systems.”
Burrows cites rising use cases that leverage deep learning algorithms to analyze video footage in real time, reducing the risk of human error in identifying and responding to security incidents. Also, AI can instantly identify and classify objects in video streams, such as vehicles, people or specific objects of interest.
“Responding quickly and taking a more proactive approach to security and safety can prevent losses, damage and accidents before they occur,” Burrows says. “And that’s particularly true across education, retail, logistics and hospitality where heads of security are looking to reduce shrink or theft, harden security and enhance health and safety.”
Integration & Compatibility
To ensure AI-based video surveillance solutions are compatible with existing security infrastructure, it will be important for systems integrators to partner with manufacturers and vendors that support open platforms for development, explains Adam Lowenstein, director of product management, i-PRO Americas, Rolling Meadows, Ill.
“Systems need to be designed to scale and adapt as technology evolves,” Lowenstein, says. “Focusing on edge-processing of AI (in-camera) keeps bandwidth and corresponding infrastructure costs low and maximizes compatibility compared with a server-only approach.”
Milestone Systems’ Fulgenico Navarro, head of applied research, provided the following key considerations for integrators working on deployments that require compatibility with existing infrastructure:
- Prioritize adhering to data standards by adopting industry-standard communication protocols and data formats. This will facilitate seamless interaction between the AI solution and the various components of the existing security system.
- Establish a common language for data exchange. This will minimize integration challenges and promote cohesive collaboration among different solutions.
- Assess the processing power, memory and connectivity of the current infrastructure to ensure its capability to support AI solutions. By doing so, organizations can minimize the need for extensive hardware upgrades and capitalize on their existing investments.
- Develop a systematic plan for transferring historical and real-time data to the AI solution. This involves careful considerations for data cleansing, format transformations, and secure migration protocols to maintain data integrity and confidentiality.
“By taking these compatibility aspects into account, systems integrators can facilitate a smooth transition, preserving valuable information stored in the existing security infrastructure while unlocking the enhanced capabilities introduced by AI-based video surveillance solutions,” Navarro says.
For integrators, a lot depends on their choice of manufacturer and whether they offer forward compatibility, Burrows explains. Price is still a barrier to adoption if end users need to replace good functioning security cameras or install additional ones dedicated to AI, such as top view cameras or angled bullet form factors.
“However, today price is no longer a big obstacle to any business that wants AI-powered video analytics,” Burrows suggests. “Easy adoption routes are now available that have brought the old barriers of cost and upgrade disruption tumbling down.”
By example, he says, for small to medium enterprises, edge AI cameras and convenient plug-in AI boxes now allow very attractive AI tools to be added to existing systems in a targeted way, delivering an instant AI upgrade that comes without upfront or ongoing licensing fees. What’s more, surveillance analytics are a force multiplier for operational efficiency.
“For larger applications, customers can adopt deep learning tools as service modules within their VMS,” Burrows adds. “These also don’t have to be expensive if they come without high ongoing license and connection fees and ensure integrators can demonstrate a low total cost of ownership.”
In general, most AI-based video surveillance solutions can work within an existing infrastructure, provided there is access to both power and network connectivity, Dunning explains. If bandwidth and cabling distance needs go beyond the current infrastructure, integrators can use a Utility Grade Infrastructure (UTG) cable to meet them. This also leaves the infrastructure well-positioned to meet future needs, she notes.
Dunning provided the following four key considerations that integrators and end users should think about when integrating AI with legacy surveillance systems:
Application — The desired application and outcomes will have the greatest impact on overall surveillance system design. For example, if the primary goal is for license plate recognition (LPR), systems require specific camera angles and resolution, which must be properly incorporated into the overall design.
Camera placement — Once the goals are confirmed, the next step is to determine camera placement, quantity, type and lighting. Certain use cases lend themselves to easy deployment within existing cameras, while others may need updated technology in order to integrate. For example, some environments — such as a school or medical facility — will require anonymity in their surveillance data capture and will need cameras to have privacy settings that blur certain areas of the image. There are also use cases that require a camera to be pointed straight downward to improve accuracy in people counting, for example.
Deployment method — There are four main types of deployments: server-based, cloud-based, edge and hybrid. Each of these have different costs, advantages and requirements that may factor into system design.
“Finally, ensuring the correct infrastructure exists to support the technology is key,” Dunning adds. “Many camera deployments can work within an existing infrastructure, provided it has access to both power and network connectivity.”
Sasikala of Teledyne FLIR also pinpoints infrastructure as key, since no security infrastructure is the same and every deployment site is unique. Therefore, she says, most AI-based video surveillance devices and systems are built for integration within different types of security infrastructure.
“During the vendor selection and solution specification stage, it is prudent for systems integrators to have early-stage technical consultations with the system providers to determine the level of integration required and the specific demands of an existing system,” Sasikala explains “This could lead to engineering collaborations and tailoring of the system specifications — for example, to support specific network protocols, cloud-based data infrastructure, to address site level restrictions to comply with cybersecurity concerns, and so on.”
Assessing Challenges & Threats
Among key challenges associated with the use of AI is contending with false positives, which are triggered by inaccurate detections. This leads to avoidable operational expense in terms of personnel costs and costs of investigative processes conducted at physical sites, Sasikala explains.
“As such, improving the accuracy in object detection and tracking determines the quality and efficacy of the AI-based surveillance system that is deployed for site surveillance,” she says. “This, therefore, requires deep neural network (DNN)-based AI to be trained on a large number of annotated targets and evaluated for precise application to different types of intrusions.”
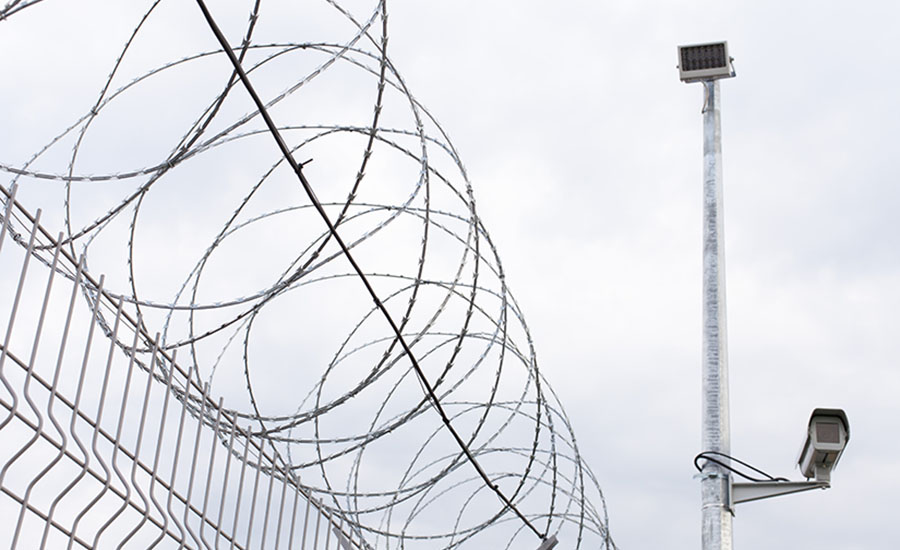
Due to elevated privacy concerns and ethical considerations when facial recognition technology is utilized within AI-based systems, Sasikala says integrators have the responsibility to ensure necessary protocols and measures for data collection, data management, storage and access by authorized personnel.
“It is also imperative that any data collected is only used for legitimate surveillance purposes within the data protection policies and guidelines laid out for the management of the security infrastructure,” she says. “If there is a proper level of transparency, a defined protocol and clear communication can be established that can go a long way to mitigate concerns around privacy rights arising out of AI-powered surveillance systems.”
Integrators and end users can mitigate privacy and ethical concerns related to AI-enabled surveillance by creating their own transparent policies, procedures and guidance for use of data to ensure AI trustworthiness and ethics, suggests Dunning of Wesco. And developing these guidelines can do more than reduce risk — it also can create a competitive advantage.
“An established proprietary framework protects their business, protects their customers, and facilitates the adoption of new technologies by streamlining decision-making,” she adds. “That transparency and clarity can provide peace of mind — both internally and to customers.”
In recent contract wins for IDIS, Burrows says facial recognition has not been used other than when deep algorithms are employed to detect a human presence, rather than a harmless animal, for example. The capabilities that customers are most excited about are tools such as people counting, which do not require any biometric data to be captured or processed. Thus, he says, the data privacy regulations that apply with these systems are no more than those the company is already acquainted with.
Systems integrators should be implementing privacy-by-design principles into the design of the system from the outset, Burrows says. These include limiting the amount of data collected, stored, and analyzed, implementing strong access controls, and using encryption to protect data in transit and at rest.
“The good news is that this can be seamless and pre-configured if integrators opt for an end-to-end solution in terms of cameras, VMS, AI software or devices, as privacy and cybersecurity measures are inherent and eliminate the chance of human error making them both easier to design, price and install,” he says.
Among a litany of considerations when utilizing AI in video surveillance, one that must be addressed is the perception of one size fits all, advises Trinh of Axis Communications.
“In the AI world, machine and deep learning techniques are powerful because they are trained on an abundant amount of data. However, abundant data isn’t enough. It’s important that it is good quality data,” he explains. “Just because a device states that it uses AI, ML or DL doesn’t mean that the performance will be guaranteed. Most of the success of AI is based on platforms that reach millions of people and have a common expected outcome. In physical security, the users are diverse and have specific needs and outcomes, making it more challenging — but not impossible.”
Also important to understand is that AI models are not intelligent — they only output what they have learned, Trinh says. Consider: A model that detects humans and vehicles will not detect other objects, such as animals, bags, boxes, etc., unless it explicitly states that it was trained on those features.
“The market confusion about what AI can do can lead to using the wrong products and services, making it harder for legitimate solutions based on AI to thrive,” he says.
Legislation is another challenge, Trinh says, as several government entities regulate AI and can be expected to continue to expand their data privacy laws. It is crucial that the security industry has a seat at the table to make sure stakeholder concerns are heard.
“We have already seen many prominent data organizations influencing legislation around their business models,” he says. “The use of AI can be very positive in our industry, and we must advocate for that in both the public and private sector.”
Norton of Milestone Systems notes that technology companies are under scrutiny from future generations and current decision-makers alike on their approach to implementing AI-driven technology. This challenge was brought to light in a global survey commissioned by Milestone Systems in 2023. Titled “Responsible Technology — A License to Operate,” the survey revealed that 85 percent of technology buyers expect responsible use of AI, video analytics and video surveillance to be a prerequisite for engaging with technology companies in the future.
“The survey underscores the significance of responsible and ethical use of AI, video analytics and video surveillance,” Norton says. “It is not merely a desirable attribute but an essential requirement for technology vendors aspiring to thrive in the future market.”